Home Check Worthiness
Identifying Check-Worthiness
Check-worthiness refers to the evaluation of a statement, claim, or piece of information to determine whether it requires fact-checking or verification. This process is essential in identifying potentially misleading, false, or unverified information before it spreads and influences public opinion. Fact-checkers, journalists, and even ordinary individuals can use the concept of check-worthiness to prioritize their efforts and focus on verifying the most important or potentially harmful claims.
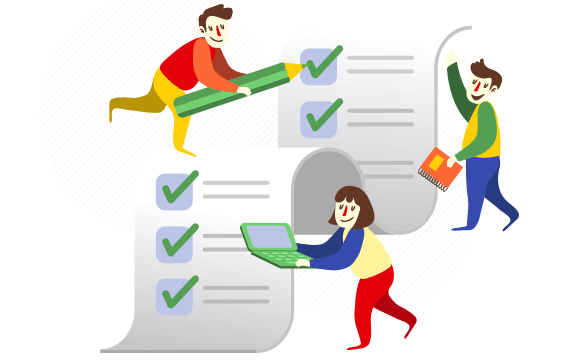
Several common factors that can help in determining
the check-worthiness of a statement or a claim:
Source:
Consider the credibility and reliability of the source making the claim. If the source has a history of spreading misinformation or has questionable credibility, the statement may be more check worthy.
Novelty:
Consider whether the claim introduces new information or contradicts previous reporting on the subject. New or unexpected claims may be more check-worthy, as they can help in identifying emerging misinformation or updates to existing knowledge.
Virality:
Assess the reach and spread of the claim on social media, news outlets, or other communication channels. If a statement is gaining significant attention or being widely shared, it may be more check-worthy, as its potential to influence a larger audience increases.
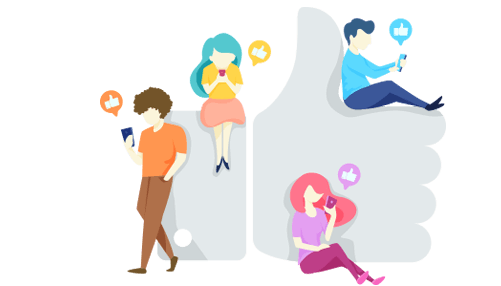
Impact:
Assess the potential impact of the claim on public opinion, policy decisions, or individual behavior. Statements that could have significant consequences if left unchecked are more check worthy.
Plausibility:
Evaluate whether the claim seems plausible or aligns with known facts and expert opinions. If a statement contradicts established knowledge or raises doubts, it may be more check worthy.
What is Check-worthiness?
Check-worthiness detection is the process of identifying and prioritizing statements, claims, or pieces of information that require fact-checking or verification. The goal is to efficiently allocate resources and efforts towards verifying the most important or potentially harmful claims. Check-worthiness detection can be performed manually by human fact-checkers or automatically AI models.
Manual
check-worthiness detection:
Fact-checkers, journalists, or individuals use their judgment and media literacy skills to assess the factors that make a claim check-worthy, such as its source, impact, plausibility, novelty, and virality.
Automatic check-worthiness detection:
NLP/ML methods are used to analyze large volumes of text or other media content to identify patterns and features associated with check-worthy claims. State-of-art approach is to train machine learning models to rank and prioritize claims, which are then used to support the work of human fact-checkers and journalists.
Automatic check-worthiness detection can help streamline the fact-checking process, enabling fact-checkers to focus on the most relevant and impactful claims. By identifying and verifying check-worthy information, fact-checkers, journalists, and individuals can prioritize their efforts to verify and debunk false or misleading claims, helping to maintain the integrity of information and promote informed decision-making.
Most notable research and initiative for check-worthiness detection include CheckThat! Lab initiative https://checkthat.gitlab.io/, which has been organizing shared tasks, creating datasets for several languages and domains.
Disinformation Detection
Disinformation is the deliberate spreading of false or misleading information with the intention of deceiving people or influencing their beliefs or actions. It is often used as a tactic by individuals, groups, or governments to manipulate public opinion or advance a particular agenda.
References
- G. Da San Martino, S. Yu, A. Barrón-Cedeno, R. Petrov, and P. Nakov, “Fine-grained analysis of propaganda in news article,” in Proceedings of the 2019 conference on empirical methods in natural language processing and the 9th international joint conference on natural language processing (EMNLP-IJCNLP), 2019, p. 5636–5646.
- J. Piskorski, N. Stefanovitch, V-A B ausier, N. Faggiani, J. Linge, S. Kharazi, N. Nikolaidis, G. Teodori, B . De Longueville, B . Doherty, J. Gonin, C. Ignat, B . Kotseva, E. Mantica, L. Marcaletti, E. Rossi, A. Spadaro, M. Verile, G. Da San Martino, F. Alam, P. Nakov, News Categorization, Framing and Persuasion Techniques: Annotation Guidelines, European Commission, Ispra, 2023, JRC132862.
- Firoj Alam, Hamdy Mubarak, Wajdi Zaghouani, Giovanni Da San Martino, and Preslav Nakov. 2022. Overview of the WANLP 2022 Shared Task on Propaganda Detection in Arabic. In Proceedings of the The Seventh Arabic Natural Language Processing Workshop (WANLP), pages 108–118, Abu Dhabi, United Arab Emirates (Hybrid). Association for Computational Linguistics.
- Dimitar Dimitrov, Bishr Bin Ali, Shaden Shaar, Firoj Alam, Fabrizio Silvestri, Hamed Firooz, Preslav Nakov, and Giovanni Da San Martino. 2021. Detecting Propaganda Techniques in Memes. In Proceedings of the 59th Annual Meeting of the Association for Computational Linguistics and the 11th International Joint Conference on Natural Language Processing (Volume 1: Long Papers), pages 6603–6617, Online. Association for Computational Linguistics.
- Dimitar Dimitrov, Bishr Bin Ali, Shaden Shaar, Firoj Alam, Fabrizio Silvestri, Hamed Firooz, Preslav Nakov, and Giovanni Da San Martino. 2021. SemEval-2021 Task 6: Detection of Persuasion Techniques in Texts and Images. In Proceedings of the 15th International Workshop on Semantic Evaluation (SemEval-2021), pages 70–98, Online. Association for Computational Linguistics.
- Boydstun, Amber E.; Card, Dallas; Gross, Justin; Resnick, Paul; A. Smith, Noah (2018): Tracking the Development of Media Frames within and across Policy Issues. Carnegie Mellon University. Journal contribution. https://doi.org/10.1184/R1/6473780.v1
- Card, Dallas, et al. “The media frames corpus: Annotations of frames across issues.” Proceedings of the 53rd Annual Meeting of the Association for Computational Linguistics and the 7th International Joint Conference on Natural Language Processing (Volume 2: Short Papers). 2015.